Artificial intelligence for sustainable insect farming
Insects are a promising new source of protein. Artificial intelligence using the vehicle of computer vision can play a key role in sustainable insect farm monitoring.
This article explores how computer vision can be used to monitor insect farms and identify potential issues early on. This could lead to more efficient and sustainable insect farming operations, with less waste and fewer pests.
Table of Contents
- Current state of sustainable insect farming?
- Importance of automation in sustainable insect farming
- How machine vision can solve the problem
- How machine vision for insect monitoring works
- Some results of the system in action
- Challenges of using machine vision for insect farming
- Future work that needs to be done in this area
Current state of sustainable insect farming
The demand for alternative protein sources has continued to rise. Hence, the importance of farming insects with an emphasis on sustainability and quality control has become increasingly more critical.
Insects have become a component of the diets of 2 billion people worldwide. Over 2,000 known insect species is reported as being consumed globally. The reasons for this growing trend are not far-fetched.
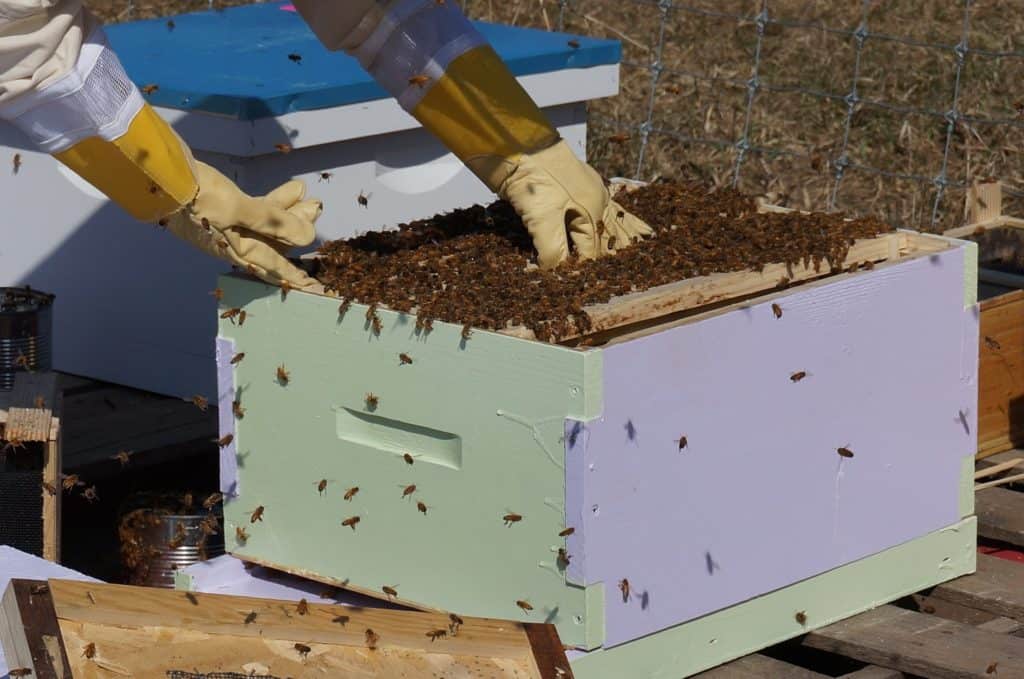
Most species of insects are very efficient in fixing biomass that are high in protein. These makes them handy and easy to breed. They require little or no processing before consumption, and they have a relatively short cycle of growth.
Apart from feeding humans, insects can also be used to feed livestock. This is because insects can consume low-grade organic waste converting it to valuable bio-products such as animal protein and lipids. These can then be used to feed and grow animals, such as fish, poultry, and pigs.
Importance of automation in sustainable insect farming
The intensive monitoring of the operations of insect breeding and production – from larvae to processing – poses unique logistical challenges for individuals and businesses who are seeking to produce high-yield, consistent harvests of insect-based products in a cost-effective manner.
In addition, insect farmers require knowledge of the growing conditions within each growing season. Therefore, artificial intelligence or an automated surveillance system that can provide essential information is needed.
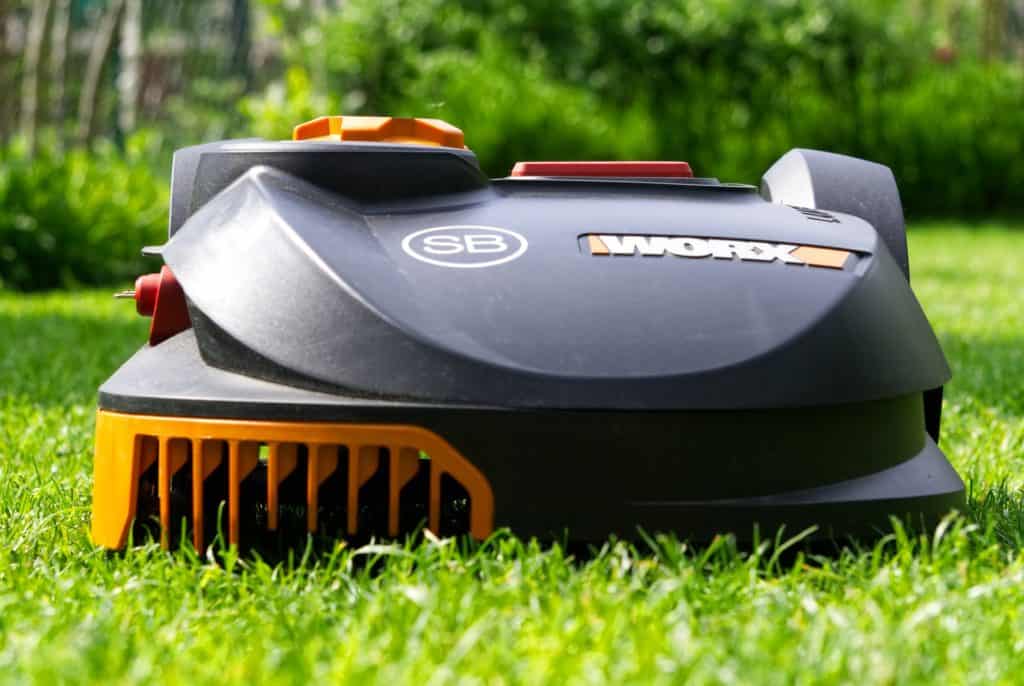
By using an automated strategy, farmers can track data points such as the number of insects, sex balance of the population, size of individual insects, temperature, humidity, and feed consumption in order to optimise their efficiency and yield while also meeting internally defined standards for high-quality insect-based proteins.
Furthermore, automation can assist in minimising waste by ensuring that resources are allocated efficiently during each production cycle.
These factors all combine to offer an efficient and sustainable way for insect farmers to produce and raise insects for food production purposes.
How machine vision can solve the problem
Machine or computer vision can be used to identify the condition and general health of farmed insects. This is in addition to the detection of any pests or diseases that might infect the colony.
With computer vision, it is possible for farmers to remotely detect changes in the environment before they become a problem. This can provide more control over the health and wellbeing of their farm.
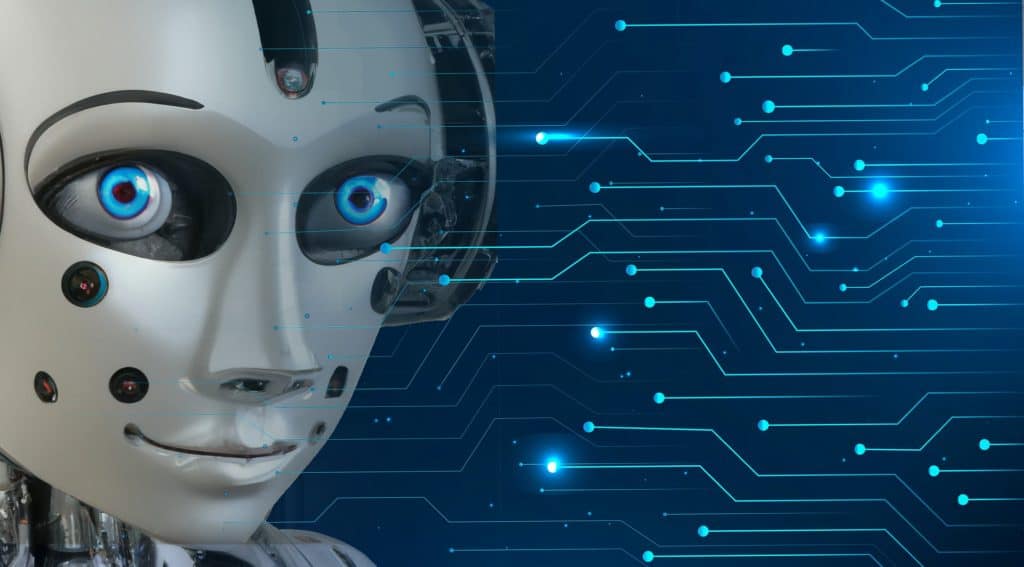
In addition, computer vision can be used for tracking the harvesting process and ensuring that insects are handled more humanely. Computer vision can revolutionize insect farming and make it easier for farmers to both monitor and protect their investments.
How machine vision for insect monitoring works
Machine vision works by using cameras and specialized computer software to identify the size, shape, and colour pattern of a given insect species.
The images are then analysed by the software to produce data about the insects’ behaviour. This can include their location, their distribution in an area, and the types of habitats they prefer.
The data generated can then be used to effectively monitor the population of a particular species. This can help conservation measures to be taken if necessary.
Some results of the system in action
In order to demonstrate the efficiency of adopting machine vision in the monitoring of insect breeding and harvesting, results have indicated that the amount, size and sex of two types of commonly farmed insects namely, the Black Soldier Fly (BSF) and crickets (Acheta domesticus) can be classified and predicted with high levels of accuracy.
Case study 1
The following are a summary of results derived from a study of the amount, size, and sex of BSF and crickets:
- The BSF larvae has been correctly classified up to and accuracy level of 87%.
- Cricket have been correctly detected up to an accuracy of 86% at an average confidence level of interval.
- High accuracy classification of cricket by sex using deep convolutional neural network has helped in the achievement of an accuracy score of up to 93%.
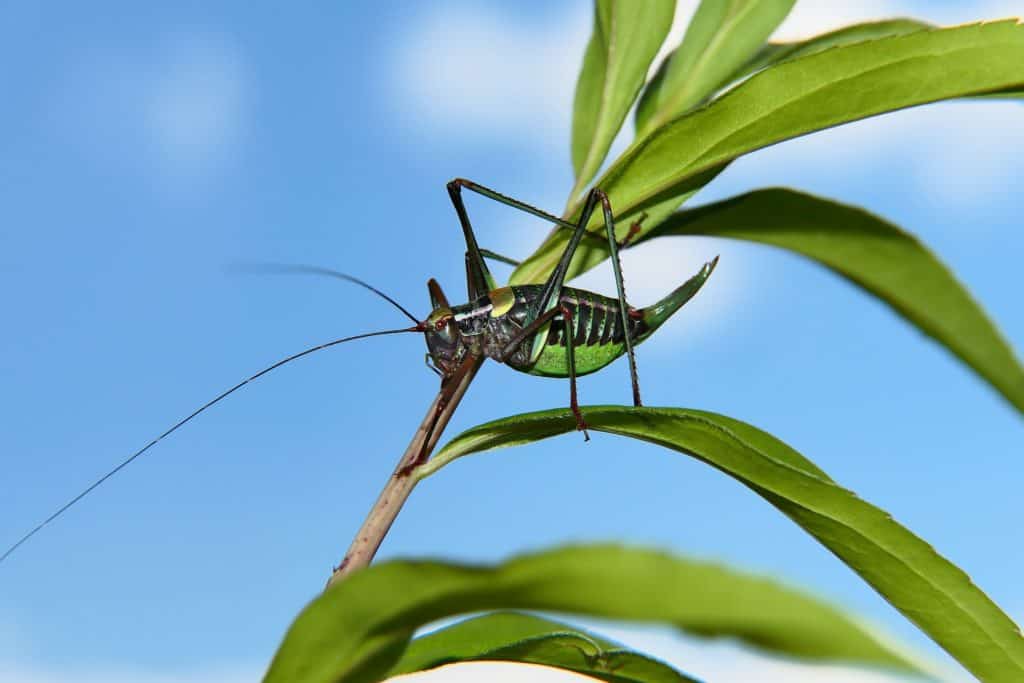
Case study 2
In a separate study, the breeding cycle of a type of beetle called Tenebrio Molitor (from the Darkling beetles’ family) was monitored.
An adult Tenebrio Molitor has a somewhat elongated body that measures about 12–18 mm in length. It is possible monitor several aspects of the life cycle of a Tenebrio Molitor. This can include stages in the development of the eggs, larvae, pupae, and beetles.
The larvae would transform into a pupae in approximately 45–60 days after 7–9 cycles of moulting. The pupae can in turn morph into beetles after the 5 days following.
The method of monitoring involved successive steps of iterative development using artificial intelligence model. This was based on synthetic image generation and application of deep learning.
Firstly, the detection and segmentation of the stages of growth of the Tenebrio Molitor (live larvae, pupae, beetles) was done. This also involved anomaly detection in the form of dead larvae and pests (Alphitobius diaperinus).
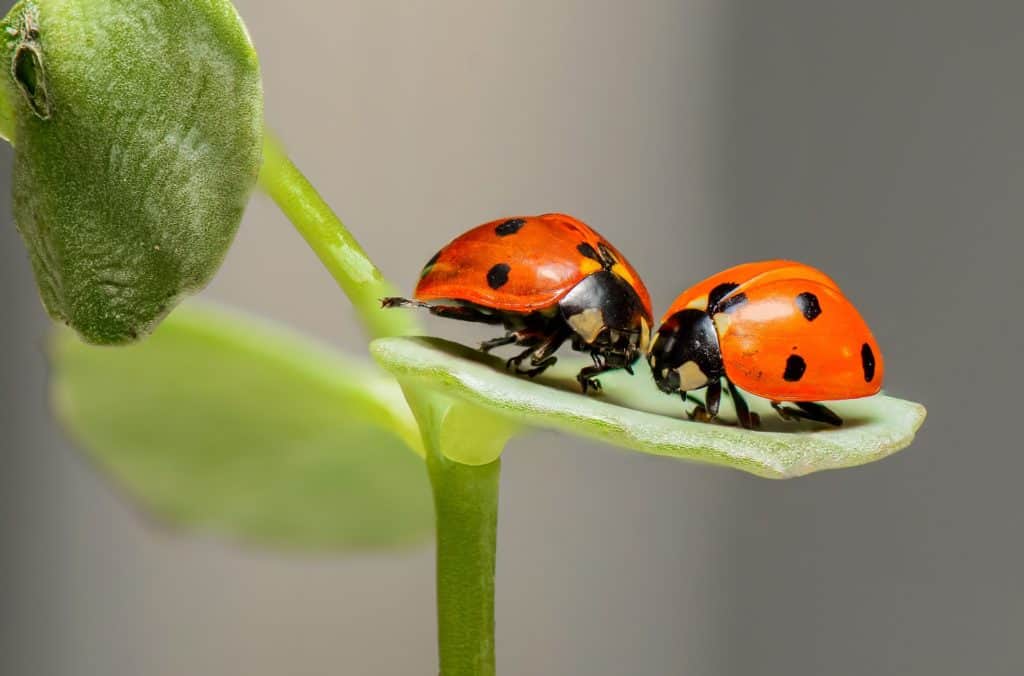
The second segmentation stage involved extracting areas that represented the densities of the feed, frass and chitin from the image, and then calculating their percentages in the whole image.
Thirdly, larvae phenotyping was done. Results indicated that these methods which Includes the development of multiclass instance and semantic segmentation of the models based on the generation of synthetic imagery and object pooling reduced the time of the iterative
improvement of machine learning models tremendously. In the process, the robustness of the models to problems such as the detection of minority class objects and dense scenes were also improved.
In addition, this method which also included an estimation of the length distribution of larvae using a box enabled effective supervision of larval growth during breeding, even though most larvae are normally considered to be invisible.
Challenges of using machine vision for insect farming
Just like any other venture at its early stages of development, there are several potential logistical and technical problems that are associated with insect farm monitoring when using artificial intelligence.
Even though the availability of cameras is very high, there is a lack of information on the algorithms that can identify even the basic parameters of insect breeding.
In this case, the objects to be identified may overlap, and the colour or texture of each instance can appear very similar to each other.
Other broader challenges can involve the inability to detect complex features such as aggregation or mating, the lack of capacity for the application of the same system to another species in a different geographical region, not being able to ensure the balancing of males and females insects, selection of individuals with desired phenotypes, longer-term observations to characterise changes in states of the insects, and the association of larval features with symptoms of disease or poor condition.
Future work that needs to be done in this area
Going forward, there is still much work to be done in using computer vision for insect farming. One area of research that needs to be explored is the development of automated systems for sorting and grading harvested insects.
This could potentially allow farmers to reduce labour costs associated with this process and increase efficiency.
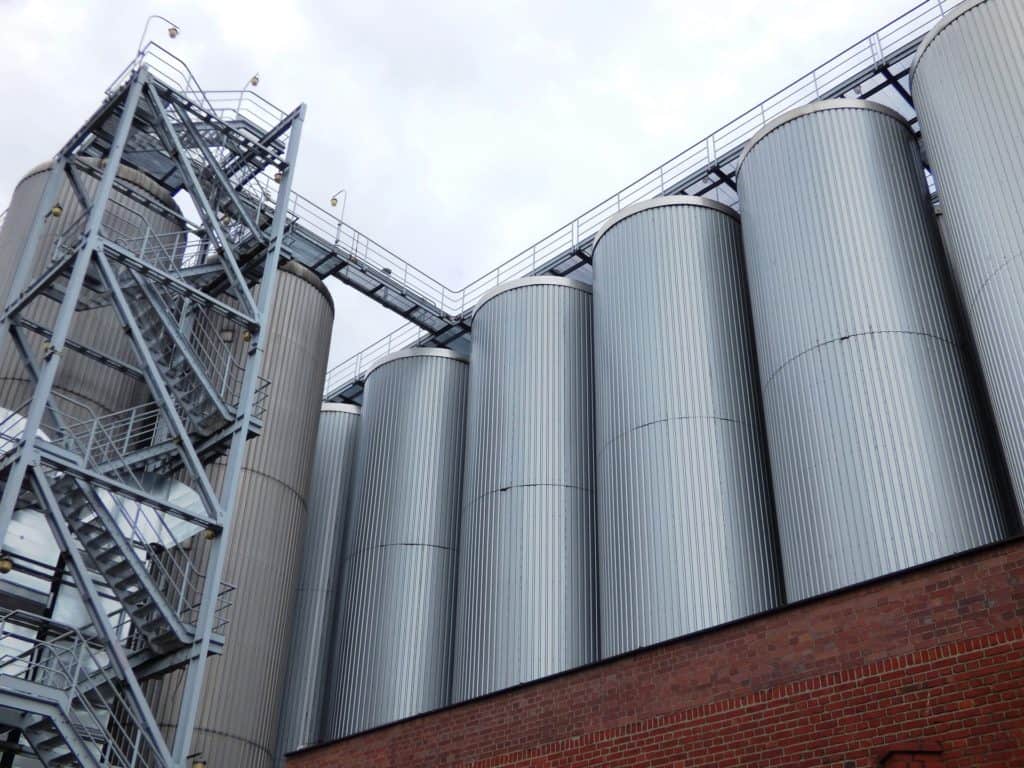
In addition, further studies into image processing techniques may help improve accuracy in detecting diseases or parasites on the insects.
Finally, developing a system for collecting data from multiple sensing technologies would enable farmers to monitor their farm conditions more effectively.
These advancements will go a long way towards making insect farming even more sustainable and efficient than it currently is.
Notwithstanding, with the continuing progress in machine vision technology, the potential applications are almost endless.
Therefore, by focusing on these areas of research, we can ensure that the future of insect farming is bright. Just ensure you hone in on a typical area of research so that the broad field of insect farming does not seem too daunting.
Final thoughts
In conclusion, sustainable insect farming is an important business for producing food and other products without harming our planet. Insects are a great source of protein and can be farmed with little land and water.
What’s more, they emit fewer greenhouse gases than traditional livestock like any farming practice, there are challenges associated with insect farming. However, with the right infrastructure and management practices in place, these challenges can be overcome.
Artificial intelligence can be used to help with monitoring insect farms, by automatically counting the number of insects in a given area or detecting signs of disease.
Machine learning methods based on computer vision are still being developed and improved, but recent results show that they have great potential for helping to ensure efficient and sustainable production from insect farms.